Have you ever wondered, what is the probability of a gamble’s outcome? This could be a casino game, a horse race, a sports bet, or some financial bet like stock, crypto, or options. In all of these situations, the probability can be estimated using the same methods.
Implied probability is an estimate of a gambling outcome’s probability based on that outcome’s payoff. This payoff is converted into betting odds which imply one probability value for that outcome. As an estimate, it can be inaccurate if the payoff depends on the opinions of the gamblers.
The implied probability is directly related to the betting odds, and around the world, there are different conventions for calculating odds. In the next section, we’ll cover how to calculate the betting odds and implied probability using a few common conventions. Let’s find out the meaning: what are the odds?
Convert Implied Probability to Betting Odds (and Back)
Table of Contents
In this section, we’ll cover what betting odds are and how to convert odds to implied probability and back. The central idea for betting odds is that for a ‘fair’ payout in a game, the probability must match the payout. With a fair game, the average value expected from repeatedly playing should be zero.
Let’s look at a simple example with a coin flip. For a fair coin, the probability of flipping heads is 50% and the probability of flipping Tails is 50%. In order for the average to equal zero, the payout for Heads should be double (+100%) and the payout for Tails losing everything (-100%).
For every other value, either the person offering the bet or the bet taker will be making money on average. With a fair payout, neither party is making money on average. The calculation is as follows:

Note: When we calculate the average here, we do not need to divide by the total number because probability always sums to 1.
From this example, we can see that the fair odds are related to the inverse of the payoff. Here I used an odds convention called Fractional Odds or ‘X-to-1’ odds. There are different conventions that people use for payoff odds, including the mathematical formulas for sports betting. I’ll go over the different conventions and explain Fractional Odds later, but for now, we have established there’s a relationship between the probability and the payoff odds.
The next time you get a hot fast tip quote the option market. I do not say that you should necessarily buy a Call, but you should at least mull over for a little the fact that there exists a group of gentlemen who seem willing to wager that this stock is NOT going to go so very high in the next 30 or 90 days after all.
Fred Schwed Jr.
In this case, we know beforehand that the real probability of the coin flip is 50%. If there is a situation where the true probability is unknown (a sports bet, horse race, stock bet, crypto trade, or financial option), the probability can be calculated from the payoff of the betting odds currently quoted by the bookie or market. In fixed-odds sports betting, the betting odds may not vary.
For example, if the payoff for a horse in a race is 1-to-1, the odds imply the speculators betting believe that the horse has a 50% probability of winning. The person taking the bet will double their money as a prize for beating the odds. If the payout is 2-to-1, the bet taker will triple their money at an implied probability of 33%.
Convert Implied Probability to Decimal Odds
In order to convert implied probability to decimal odds, you only need to take the reciprocal of the probability.
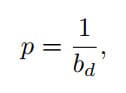
where p is the implied probability, and b is the payout measured in decimal odds. This convention is primarily used in Canada, Australia, New Zealand, and Europe (minus the UK). Decimal Odds are also known as European Odds, Continental Odds, or X-for-1 Odds. [1]
The idea surrounding this convention is that the person taking the bet needs to first risk their money. As a result, the odds value reflects the total amount which is given back to the bet taker. If the Decimal Odds are 2, the bet taker will double their bet if that outcome happens.
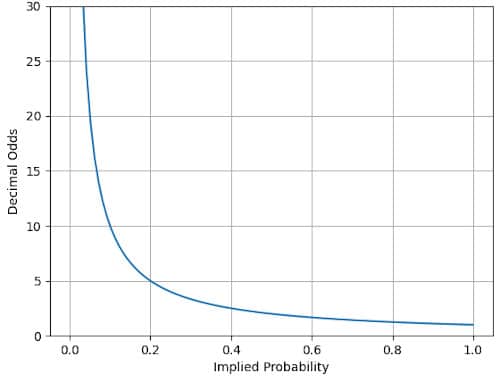
With Decimal Odds, it is easy to see the inverse relationship between the payout and probability. For high payouts, the outcome is expected to be low probability. For low payouts, the outcome is expected to be high probability.
Decimal Odds are the convention used in the Potion Analytics THICC repository. Check out more about the Potion Protocol here.
Convert Implied Probability to Fractional Odds
In order to convert implied probability to fractional odds, you take the Decimal Odds value and subtract 1. The formula is as follows:
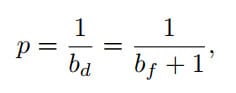
where p is the implied probability and b is the payout measured in fractional odds. This convention is primarily used in the UK and in Ireland, though it is also often used in horse racing bets. Fractional Odds are also known as UK Odds, British Odds, Traditional Odds, or X-to-1 Odds. [1]
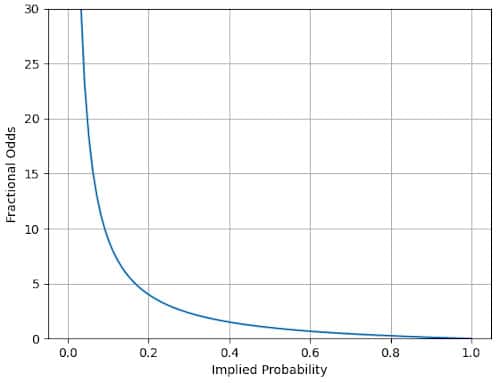
For this Odds convention, the amount does not include the original amount wagered. For this reason, Fractional Odds differ from Decimal Odds by 1 (the winning amount, minus the original bet). If the Fractional Odds are 1, the bet taker will double their money if that outcome happens.
Convert Implied Probability to Moneyline
Moneyline Odds are sometimes known as American Odds and are often used for sports betting. Just like other unit systems we use in the US, it’s somewhat unique. For Moneyline Odds there are separate formulas for positive and negative numbers. [1]
For positive numbers, the formula is simply 100 times the Fractional Odds. The Moneyline Odds represent the amount paid out for a $100 bet on the outcome. In other words, if the value is +200, the person making the bet will win $200 for a $100 bet. The formula is:
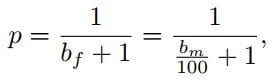
where p is the implied probability and b is the payout measured in Moneyline Odds. For negative Moneyline values, the formula is a different one because the value represents the amount needed to risk in order to win $100. The formula is as follows:
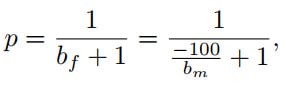
where p is the implied probability and b is the payout measured in Moneyline Odds. For example, if the Moneyline Odds are -200, the Fractional Odds are 0.5 and the implied probability is 66.6%.
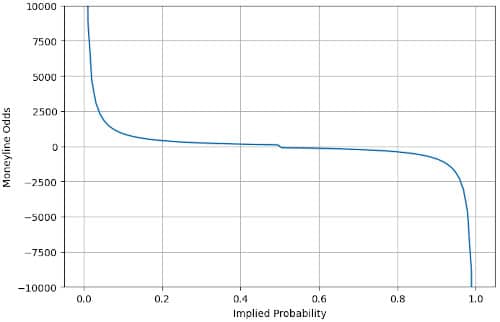
Convert Implied Probability to Return
While it is not technically an Odds convention, we can convert from the return on a bet to implied probability. The return is simply the Fractional Odds multiplied by 100% (also the positive formula for Moneyline Odds). The formula is:
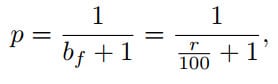
where p is the implied probability and r is the return.
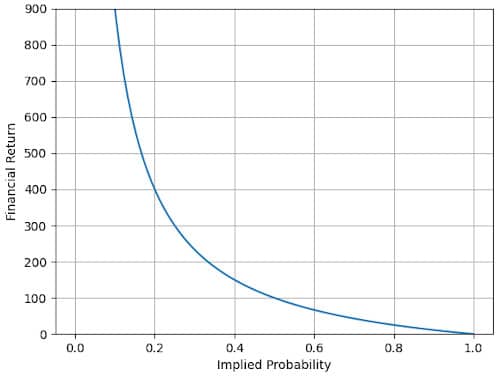
Miscellaneous Odds and Ends
Other less popular Odds conventions include Hong Kong Odds, Malaysian Odds, and Indonesian Odds. There are a few common definitions and expressions surrounding betting odds. [1]
- True Odds: The actual unknown (and objective) odds. The True Odds are often different from what the bet takers and bookmakers believe.
- High Odds: The high payoff for an unlikely and low probability outcome.
- Low Odds: The low payoff for a likely and high probability outcome.
- Odds On: The payoff for the most likely and highest probability outcome.
Implied Probability | Fractional Odds | Decimal Odds | Moneyline Odds | Return |
---|---|---|---|---|
0.01 | 99 | 100 | +9900 | 9900% |
0.1 | 9 | 10 | +900 | 900% |
0.2 | 4 | 5 | +400 | 400% |
0.3 | 2.33 | 3.33 | +233 | 233% |
0.4 | 1.5 | 2.5 | +150 | 150% |
0.5 | 1 | 2 | +100 | 100% |
0.6 | 0.67 | 1.67 | -150 | 67% |
0.7 | 0.43 | 1.43 | -233 | 43% |
0.8 | 0.25 | 1.25 | -400 | 25% |
0.9 | 0.11 | 1.11 | -900 | 11% |
0.99 | 0.01 | 1.01 | -9900 | 1% |
Implied Probability in Options
Now that we know how to calculate the implied probability for gambling games and sports bets, let’s move on to how it is calculated for stocks, crypto bets, and the options market. Implied probability is a useful tool for evaluating the sentiment and beliefs of the market.
In contrast to using the historical price data of the asset, using implied probability can incorporate the uncertainty of future information like earnings announcements for stock, a decision for a DAO or a crypto fork, or an upcoming event like an election or the release of economic data. Unlike using historical data, the implied probability is not only backward-looking.
There are two common methods for using options to create an implied probability distribution and I’ll cover them both.
Implied Probability of a Butterfly Spread
Using a Butterfly Spread is the simplest method of calculating the implied probability from option prices. A Butterfly Spread consists of 3 legs.
First, buy a Call at strike $X, sell two calls at strike X+Y, and finally buy a call at strike X+Y+Z. Here’s an example at the money on SPY using the closing prices on 4/27/2022:

The payout function of the spread at expiration looks like a triangle:
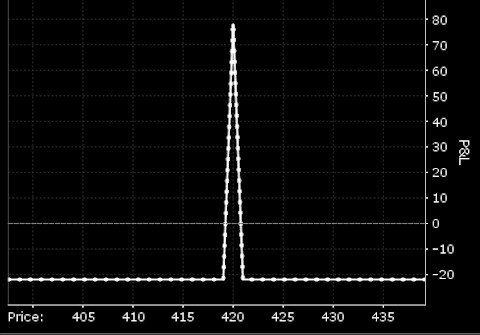
The triangle will move slightly up or down vertically depending on where within the Bid-Ask spread the trade would be filled.
By examining the payoff of the Butterfly Spread, the odds and the implied probability can be calculated. If the price at expiration is between the two strikes X and Z, there is a payout to the spread buyer. If the price at expiration is outside the strikes X and Z, the spread costs the spread buyer a fixed premium.
The positive payout can be divided by the premium cost to produce the Decimal Odds of the spread. Alternatively, one of the other betting odds conventions can be used. With the payoff odds calculated, it can be converted into implied probability using the formulas in the previous sections of this post.
This produces a measurement of the probability in a ‘bin’ between the strikes X and Z. Finally, if we iterate over all the strikes currently available in the options market, a probability value for every bin can be derived. This creates a probability distribution at the expiration date. Alternatively, that distribution can have the discount factor applied. [3] With a constant interest rate r:
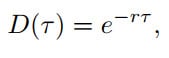
where tau is the time period to expiration in years if the interest rate r is an annualized interest rate. Putting the bins together, we get a full distribution:
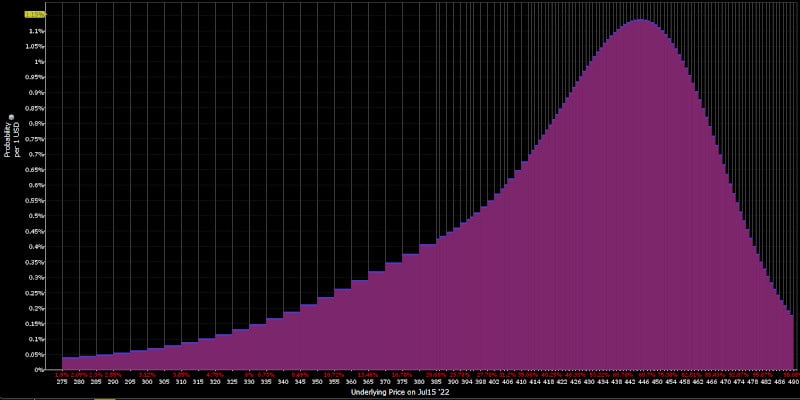
What is the Breeden-Litzenberger Formula?
The previous result is fantastic and computationally very easy. There are however some trade-offs to the method which I’ll discuss. There is also an alternative method with trade-offs that uses a different formula.
The Breeden-Litzenberger Formula links the implied probability distribution to the second derivative of Call prices with respect to the strike. Since probability must be positive, the second derivative is always positive. This results in the convexity of the option price.
The formula is as follows:
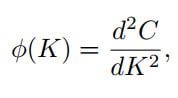
where phi is the probability, K is the strike, and C is the price of a Call option. [2] In theory, with this formula, the Call prices at each strike can be observed and computed directly. In practice however, the noise present in real-world data will cause problems.
Since there is a restriction that the probability must always be positive, the second derivative must also be positive. If the real-world noise causes the second derivative to be negative, it can produce negative implied probabilities. In addition, the fact that options are not traded on every strike prevents accurate calculation of the derivative in some cases.
Often, this real-world data is first filtered or smoothed in order to prevent this. Removing the noise is not a trivial task however and is outside the scope of this post. If care is not taken to respect the boundaries present on the price of an option, arbitrage opportunities can be introduced in the prices by the filter algorithm. [4]
Disadvantages of the Butterfly method:
- Requires many strikes to produce accurate probability everywhere
- Sensitive to noise in input data
- Probability values may not be monotonic
Disadvantages of the Breeden-Litzenberger method:
- Requires the input data to be filtered or smoothed
- The filtering method can introduce arbitrage if the wrong method is used
- More computationally intensive
How Do You Use Implied Probability?
Knowing the implied probability is useful in a range of different applications. As a result, there are several ways implied probability can be used. I’ll cover a few of the common ones.
Implied probability is used to understand the opinions of speculators in a gambling game or the market in investing and trading. The people placing bets are incorporating the information they have about possible future events, and as a result implied probability can reflect information about events like elections or earnings announcements.
The Implied Probability distribution can be propagated into the future. Want to find out how? Check out my post about using convolution!
Some speculators use the implied probability to place their bets when they believe the market to be incorrect. For example, a bullish speculator might choose to wait until the implied probability shows the market believes an asset’s price will drop to place their bet. Since ‘everyone’ is betting that the asset will go down, the premium cost of betting up with options is cheaper than it would be otherwise.
Others might believe that the market is mostly efficient at incorporating future information and choose to try to bet in the same direction as the implied probability distribution.
References
- Cortis, Dominic. “Expected values and variances in bookmaker payouts: A theoretical approach towards setting limits on odds.” The Journal of Prediction Markets 9.1 (2015): 1-14.
- Breeden, Douglas T., and Robert H. Litzenberger. “Prices of state-contingent claims implicit in option prices.” Journal of business (1978): 621-651.
- Bahra, Bhupinder. “Implied risk-neutral probability density functions from option prices: theory and application.” (1997).
- Fengler, Matthias R. “Arbitrage-free smoothing of the implied volatility surface.” Quantitative Finance 9.4 (2009): 417-428.